IDIOSYNCRATIC RISK, STOCK RETURNS AND INVESTOR SENTIMENT
1Assistant professor, Department of Finance, MingDao University ChangHua, Taiwan
2Associate professor, Department of Marketing and Distribution Management, Wufeng University, Chiayi, Taiwan
3Department of Finance, MingDao University, ChangHua, Taiwan
ABSTRACT
A growing number of studies show that idiosyncratic risk is positively related to stock returns. However, the results of such works are not consistent with each other. Since the weighting function of prospect theory is not linear, this implies that when investors make decisions under uncertainty they use a dual-classification process. This paper thus argues that applying a more appropriate research method could help to clarify the relationship between idiosyncratic risk and stock returns. This paper applies a Panel Smooth Transition Regression model to conduct an empirical study. The results show that idiosyncratic risk is positively related to stock returns, as is investor sentiment. For a given idiosyncratic risk, retail investors with low sentiment require lower stock returns than investors with high sentiment.
Keywords:Investor sentiment Panel threshold regression Idiosyncratic risk Stock returns. Threshold value Dual-classification process.
ARTICLE HISTORY: Received:30 June 2017. Revised:16 August 2017. Accepted:22 January 2018 Published:20 July 2018.
1. INTRODUCTION
It is generally accepted with regard to the capital asset pricing model (CAPM) that the level of idiosyncratic risk (IR) will decrease when the number of assets in a portfolio is increased. However, recent empirical evidence shows that this is not always true (Goyal and Santa-Clara, 2003 ; Bali et al., 2005
; Dennis and Strickland, 2005
; Wei and Zhang, 2005
; Malkiel and Xu, 2006
; Bali and Cakici, 2008
; Guo and Savickas, 2008
; Fu, 2009
; Jiang et al., 2009
) and that IR may even increase when the number of assets rises (Rajgopal and Venkatachalam, 2011
). Since these anomalies are not well explained by classical financial theory, e.g., the CAPM model, many researchers have looked for answers from a behavioral viewpoint. For instance, Fama and French (1992;1993
) state that stock returns are affected not only by fundamental and economic factors, but also by traders’ behaviors. Campbell et al. (2001
) gather monthly data from 1962 to 1997, and find that IR is one of the main components of aggregate risk. Their results also show that the overall aggregate risk did not change significantly during the sample period, but IR did rise significantly over the same time. They further separate the sample years into bullish and bearish periods to observe the changes in IR under different scenarios. Their results show that IR in bull markets tends to be higher than in bear markets, consistent with the results obtained by Brandt et al. (2010
). The relationship between IR and stock returns may thus vary with market conditions.
The stock price is determined by the behaviors of noise traders and rational investors (Shiller, 1984 ; Shleifer and Summers, 1990
). Noise traders are prone to over- or under-react to market news. As a result, changes in stock prices caused by noise traders will lead to the condition that IR will not necessarily decrease when the number of assets in a portfolio is increased (Rajgopal and Venkatachalam, 2011
). Based on the assumption of an efficient market, DeLong et al. (1990
) find that noise traders’ shareholdings will change corresponding to their unpredictable beliefs with regard to the market. Based on their personal beliefs, some investors may reduce their shareholdings or even completely leave bear markets. Therefore, the behaviors of noise traders affect the changes in IR for the overall market.
In a study on predicting stock price movements, Brown and Cliff (2004 ) conclude that institutional investor sentiment is one of main factors that can be used as a measure to identify investors’ predictions with regard to future prices. Baker and Wurgler (2006
) conclude that institutional investors with high sentiment tend to either neglect negative information or overreact to positive information, and this then results in overvalued prices. By contrast, institutional investors with low sentiment tend to cause undervalued prices. Therefore, institutional investor sentiment will affect stock prices and thus change the expected returns.
Whether IR is positively related to stock returns still remains unclear. In addition to finding that IR is positively related to stock returns, Guo and Savickas (2008 ) also find that portfolios with high IR tend to have low stock returns in the US and UK stock markets. Some researchers argue that this is because the appropriate proxies for IR have still not been found. Since the weighting function of prospect theory (Kahneman and Tversky, 1979
) is not linear, this implies that investors who make decisions under uncertain conditions use a dual-classification process. Current empirical studies of this issue either apply cross-sectional or time-series analysis, which adopt a unit-classification process, and the results obtained in this way could be questionable. Meanwhile, current studies of investor sentiment (IS) focus on the relationship between institutional IS and changes in expected returns, and the issue of whether retail IS will change these returns is ignored. These reasons thus motivate the current paper’s effort to apply a dual-classification analysis method, and to explore whether retail investors sentiment changes the expected returns.
This paper applies panel smooth transition regression analysis to examine whether studies of IR should adopt a dual-classification process, with could enrich the literature on the research methods used to assess IR. This paper also aims to identify whether the relationship between IR and stock returns varies with retail IS, and the results should provide more convincing evidence as to whether IR are positively related to stock returns.
The empirical results obtained in this paper add at least three interesting findings to IR studies. First, the panel smooth transition regression analysis identifies the existence of dual-classification conditions. Therefore, a research method which deals with this dual-classification process should be used in studies of IR and stock returns. Second, IR is positively related to stock returns. Third, retail IS is positively related to stock returns. These results can help investors to predict the relationship between IR and stock return by using IS.
The rest of this paper is organized as follows: Section 2 discusses the literature, while Section 3 then describes the data and empirical method used in this work. Section 4 presents empirical results, and Section 5 then concludes this paper.
2. LITERATURE REVIEW
2.1. Idiosyncratic Risk and Stock Returns
A stock portfolio consists of systematic risk and idiosyncratic risk. Since retail investors are usually unable to hold enough number of stocks in a portfolio to diversify risk, most of their portfolios have high levels of IR. Moreover high IR does not only appear in retail investors’ portfolios, but is also often found in those of institutional investors. For instance, Malkiel and Xu (2006 ) find that institutional investors have a better ability to manage risk, and thus tend to pay less attention to IR. These findings are robust across the New York Stock Exchange (NYSE) and National Association of Securities Dealers Automated Quotations (NASDAQ) markets. Institutional investors’ portfolios thus do not necessarily have less IR than those of retail investors, and Dennis and Strickland (2005
) state that the recent increase of IR in the US markets could be because institutional investors have increased their shareholdings.
The question of whether stock returns are affected by IR has attracted the attention of many researchers. Using a constant weight method to calculate the IR index, Goyal and Santa-Clara (2003 ) find that the stock returns are positively related to IR. However, other researchers find different results with regard to the relationship between IR and stock returns. Guo and Savickas (2008
) use data from G7 stock markets to study the relationship between IR and stock returns, and find that the stocks with high IR tended to have low stock returns in the US and UK stock markets. Fu (2009
) uses EGARCH (1,1) to estimate the IR, and finds that it has a positive relationship with expected stock returns. However, after eliminating penny stocks, Jiang et al. (2009
) provide empirical evidence that stock returns are negatively related to IR. Bali and Cakici (2008
) establish portfolios by buying stocks with high IR and selling stocks with low IR to examine the relationship between IR and expected returns. Their results show that the relationship between IR and expected returns is not significant.
By retrieving firm-level data from the CRSP database, including firms traded on the NYSE, AMEX, and Nasdaq from 1962 to 1997, Campbell et al. (2001 ) examine whether IR is impacted by the business cycle. Their results show that the IR tends to be higher in bear markets, but lower in bull ones.
2.2. Investor Sentiment
In a study of market efficiency, DeLong et al. (1990 ) first note that noise traders affect market efficiency. They then find that investor sentiment (IS) is one of main factors related to changes in stock returns. Brown and Cliff (2004
) further separate investors into institutional and retail investors to examine whether changes in stock returns can be explained by IS. They find that the sentiment of both institutional and retail investors is related to stock returns. Furthermore, Fisher and Statman (2000
) indicate that when investors experience high sentiment, they tend to overweigh the stock price. As a result, the IS leads to negative stock returns in the next period. By contrast, when investors experience low sentiment they tend to underweigh the stock price, and thus IS leads to positive stock returns in the next period. Consequently, changes in IS lead to changes in stock returns under different market conditions.
Baker and Wurgler (2006 ) develop a sentiment index with six proxies, which are the number of initial public offerings (IPOs), the average first-day returns of IPOs, the dividend premium, the closed-end fund discount, the New York Stock Exchange (NYSE) turnover, and the equity share in new issues, to discuss the cross-sectional relationship between IS and stock returns. Their findings indicate that when investors experience low sentiment, companies which are small cap, high volatility, newly listed, fast growing, and have not yet distributed dividends tend to have higher stock returns in the next period. Chou et al. (2007
) apply a number of indexes, including the turnover ratio, stock initial offering ratio, margin trading and short selling ratio as sentiment indicators to examine whether IS is related to stock returns in Taiwan. Their findings reveal that only the market turnover ratio could fully explain the stock returns in this context, and that IS is negatively related to next period stock returns. Tsai et al. (2009
) use Principal Components Analysis to construct sentiment indicator, and also find that IS is negatively related to the next period stock returns. In sum, the results of the studies outlined above cannot answer the question of whether stock returns are affected by idiosyncratic risk. The results of these works are affected by changes in index composition, methodology used in the analysis, and the sampling approach. From a methodological viewpoint, the results vary due to applying either cross-section or time-series analysis (Goyal and Santa-Clara, 2003
; Wei and Zhang, 2005
; Ang et al., 2006
; Malkiel and Xu, 2006
; Bali and Cakici, 2008
). Therefore, this work utilizes the PSTR approach to examine whether the relationship between IR and stock returns is affected by IR.
3. METHODOLOGY
3.1. Utility Theory and Implications for Methodological Design
In consumption-based utility theory, the major component of stock volatility initiates from changes in risk aversion that are caused by consumption. These changes are driven by previous stock market movements and news about market dividends (Barberis and Huang, 2009 ). Nonetheless, Barberies et al. (2001
) report that dividends are weakly correlated with consumption. Research thus implies that by interpreting utility from changes in the value of final wealth by using the concept loss aversion, it is possible to better understand the features of decision-making under uncertainty (Kahneman and Tversky, 1979
; Barberis and Huang, 2009
). This view point is commonly applied in studies. For Instance, Lee et al. (2011
) apply the concept of loss aversion to develop asset management strategy with portfolio insurance.
Prospect theory utilizes a value function and weighting function. The stylized weighting function is a probability weighting function. Gonzalez and Wu (1999 ) report that people do not see probabilities linearly, as they tend to overweigh small probabilities and underweigh large ones. They find that a probability function is inverse-S-shaped, being concave for low probability and convex for high probability. Since this probability distortion exists in decision-making, we can infer that when investors make decisions under uncertainty they use a dual-classification process. This paper therefore argues that applying a more suitable research method could help to clarify the relationship between idiosyncratic risk and stock returns. As such, it utilizes Panel Smooth Transition Regression Analysis in its empirical study.
3.2. Data
This study includes stock returns, IR, IS, the Fama and French (1993 ) three-factor model variables, as well as some control variables. All data is retrieved from the Taiwan Economic Journal (TEJ) database on an annual basis. The data for the Fama and French three-factor model is retrieved from the Taiwan Securities Market Multifactor Database of the TEJ on a daily basis. Since the accounting principles used by the financial industry are different to those used by other companies, we exclude banking and financial companies in this study. Meanwhile, since this study needs complete data for the panel data analysis, we exclude all delisted companies and those with incomplete data. The final dataset thus consists of 656 listed companies over the period from the 1st of January 2006 to the 31st of December 2013.
3.3. Variables Measures
3.3.1. Idiosyncratic Risk
This study applies the Fama and French (1993 ) to estimate the idiosyncratic risk ( IRi,t ) of the year r for firm i. This estimation is as follows:
3.3.2. Investor Sentiment
Investor sentiment is usually observed by proxies, and these can be either direct or indirect. The direct approach uses measurements from questionnaires. For instance, Shiller et al. (1996 ) obtain a sentiment index by asking institutional investors their opinions of US and Japanese stock markets every half year.
However, some studies question the credibility of institutional investors, and thus the benefits of obtaining an IS proxy using questionnaires. A number of researchers believe that an IS proxy can be obtained using only market data, and such proxies include the closed-end fund discount, market returns after IPO, trading volume, stock turnover ratio, number of stocks offerings, securities financing change rate, call and put option trading volume ratio, odd-lot trading ratio, open-ended fund redemption and weather conditions.
By applying the weekly data retrieved from the New York Stock Exchange (NYSE) and American Stock Exchange (AMEX), Conrad et al. (1994 ) find that the turnover ratio is positively related to investor sentiment. They show that when investors have high sentiment they are prone to trade stocks more frequently. As a result, high sentiment leads to a high trading volume. Baker and Stein (2004
) carry out a similar study by examining noise traders, and like Conrad et al. (1994
) their results show that when such traders have high sentiment, they are prone to trade more frequently. The sentiment of noise traders is thus positively related to the turnover ratio.
The studies reviewed above show that changes in IS will lead to changes in turnover ratios. The turnover ratio can thus be used as an indicator of investor sentiment. In this study, the turnover ratio ( Turnr,i,t) is defined as company i’s trading volume in month t divided by the number of outstanding shares. In order to more clearly assess how noise traders’ IS relates to stock returns, we further separate the turnover ratio into institutional and retail investors’ turnover ratios. The turnover ratio of institutional investors is defined as company i’s institutional investor trading volume in month t divided by the number of outstanding shares.
The turnover ratio of retail investors (RSi,t) is defined as company i’s market turnover ratio minus company i’s institutional investor turnover ratio. Since retail investors usually exhibt herding behaviour and tend to be by rumours, they are usually regraded as noise traders, and so their turnover ratio could be an aggregate of the noise traders’ turnover ratio. Because the aim of this work is to observe whether the noise traders’ IS changes the stock returns, we thus use the turnover ratio of retail investors as a proxy of noise traders’ IS for the empirical study.
3.3.3. Control Variables
Firm size, book-to-market ratio and beta coefficient are the control variables. Firm size is determined by the company’s annual market value. The book-to-market ratio is obtained by dividing the market value of a firm by its book value. Finally, we use the Capital Asset Pricing Model to calculate the beta coefficient.
3.4. Panel Smooth Transition Regression Analysis
The advantages of performing panel smooth transition regression analysis in this study are as follows: first, it allows heterogeneity to exist for each company, and for this heterogeneity to still exist as time passes. Second, it can control for some time independent cross-sectional factors. Third, it is good at dealing with dual-classification conditions.
Normally m=1 or m=2 in panel smooth transition regression analysis is sufficient to assess parameter variation Gonzalez et al. (2005 ). Specifically, in the case of m=1, this means that there are two types of classifications and the transformed variable is monotonic. In the case of m=2, this means that there are three types of classifications and the number of transformed variables is two. Prospect theory states that investor decision-making under conditions of uncertainty is a dual-classification transforming process. Therefore, the results obtained by panel smooth transition regression analysis can more accurately reveal certain market phenomena, and this approach is applied here to examine whether the relationship between IR and stock returns varies with the retail sentiment (RS).
The analysis model is as follows:
4. EMPIRICAL RESULT
4.1. Descriptive Statistics and Unit Root Test
Table 1 presents the summary statistics for all variables. The empirical results show that the abnormal return of stock is 5.63% and its standard deviation is 53.30%. The IR is 30.31% and its standard deviation is 10.31%. None of the variables meet the normality assumption, as examined using the Jarque-Bera test criterion. Comparing these results to those of recent studies, in which the institutional IR ranges from 1% to 16.86% (Guo and Savickas, 2008 ; Fu, 2009
; Jiang et al., 2009
) we find that the IR in the Taiwanese stock market is markedly higher than in European and American stock markets. The data from the Taiwanese stock market is thus a good sample to observe the phenomenon of IR.
A unit root test has to be performed before conducting the analysis. This enables us to ensure the results will not be bounded by spurious autocorrelations, which often occur in stock returns analyses. The tests used include: (1) the PP-Fisher Chi-square test (Phillips and Perron, 1988 ) (2) the LLC test (Levin et al., 2002
) and (3) the IPS test (Im et al., 2003
) with the results presented in Table 2. We find that all statistics are significant at the 1% level, and thus there are no spurious autocorrelations in the final results.
Table-1. Univariate analysis
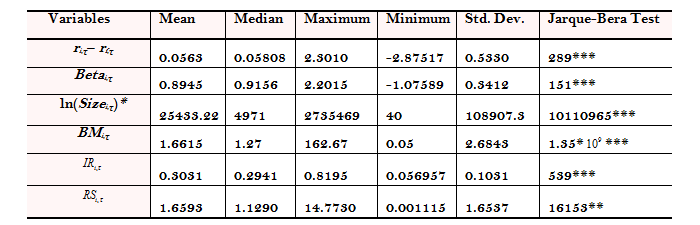
Table-2. Panel Unit Root Testsa
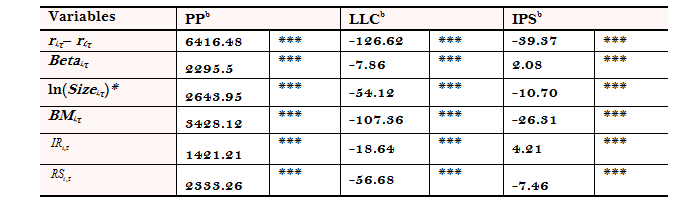
Notes: aIn order to avoid any potential spurious regressions, three test statistics are applied to determine whether the variables in the model are stationary; these are: (i) the PP-Fisher Chi-square test (Phillips and Perron, 1988 ) (ii) the LLC test (Levin et al., 2002
) and (iii) the IPS test (Im et al., 2003
). H0: the variable is a unit root; H1: the variable is not a unit root.
b*** indicates statistical significance at the 1% level.
4.2. Panel Regression Analysis
Table 3 reports the results obtained by the panel smooth transition regression analysis. Panel A in Table 3 shows that IR is positively related to stock returns. It also indicates that when stocks have high IR, investors intend to require high risk premiums. When portfolios consist of stocks with a large firm size or high book-to-market ratios, investors will also require high risk premiums. These findings are consistent with the results obtained by Fu (2009 ).
Panel B in Table 3 shows that IS is positively related to stock returns. That is, when retail investors have high sentiment, they tend to request high returns. Finally, Panel C shows that IR is positively related to stock returns, as is noise traders RS.
Table-3. Panel Regression Analysis
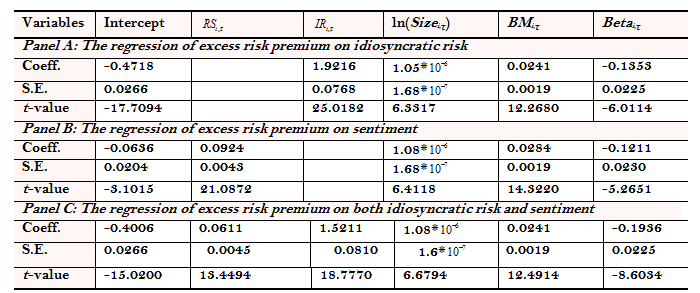
Note: RS denotes the retail investor sentiment. IR is the idiosyncratic risk. ln(Size) denoting the market value and BM denoting the book-to-market ratio are the control variables. Beta is the market factor. The *, ** and *** indicate the statistically significant at the 10%, 5% and 1% level, respectively.
4.3. Determining the Number of Thresholds
Before starting the panel regression analysis it is necessary to examine whether the dual-classification process really exists, and thus we conduct a homogeneity test. This includes the likelihood ratio test, Wald test and Fisher test, and Table 4 shows the results. According to data shown in Panel A in Table 4, we can see that at least one threshold exists, and thus whether the IR is positively related to stock returns in at least two scenarios should be examined.
We further identify the number of thresholds for analysis. The data in panels B and C in Table 4 shows that the null hypothesis of two thresholds is not rejected at a 1% significance level, and thus this study applies two thresholds for the panel regression analysis. We apply MSE, AIC, and SBC rules to identify the number of transition functions, and the results show that one transition function is enough to meet the needs of this study.
The results in Table 5 show there are two thresholds, -1036% and 36.42%. Theoretically, this paper should apply two thresholds to discuss the empirical results. However, we apply only one of them in discussing the results, as the RS of all the samples are in the range from 0.1% to 1477%. Since no company is in the range from -1036% to 0.1%, we can ignore the threshold of -1036%. Therefore, this paper uses only the threshold of 36.42% to discuss whether IR is positively related to stock returns in scenarios in which the noise traders’ IS is below 36.42% and above 36.42%.
Table-4. The tests for homogeneity
Variables | Test for Homogeneity | Test for No Remaining Homogeneity | |
(1) a | (2) b | (3) c | |
Likelihood ratio test | 286.16 | 25.93 | 10.31 |
P-value | 0.00 | 0.00 | 0.03 |
Wald test | 278.52 | 25.87 | 10.30 |
P-value | 0.00 | 0.00 | 0.03 |
Fisher test | 21.39 | 5.67 | 2.25 |
P-value | 0.00 | 0.00 | 0.06 |
Notes:aIn the test for homogeneity, H0 is the linear model, whilst H1 is the panel smooth transition regression (PSTR) model with at least one threshold variable r=1.
bIn the test for no remaining homogeneity, H0 is the PSTR model with r = 1, whilst H1 is the panel smooth transition regression (PSTR) model with at least one threshold variable r = 2.
cIn the test for no remaining homogeneity, H0 is the PSTR model with r = 2, whilst H1 is the panel smooth transition regression (PSTR) model with at least one threshold variable r = 3.
Table-5. Parameter estimates of the Panel Smooth Transition Regression model
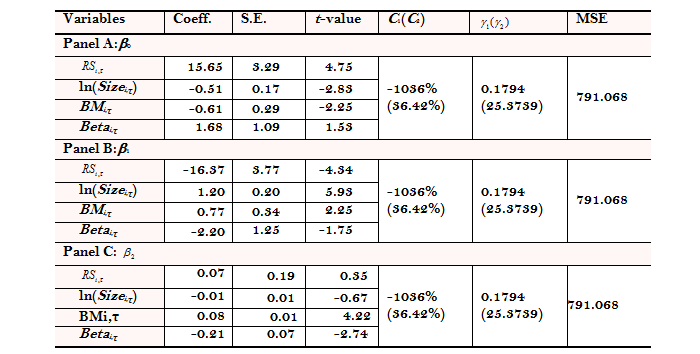
Note: RS denotes the retail investor sentiment. ln(Size) denoting the market value and BM denoting the book-to-market ratio are the control variables. Beta is the market risk factor.
Table 6 shows the results of the analysis. It indicates that IR is negatively related to the risk premium. However, the marginal risk premium is significantly positive in the first range. This can be seen as evidence that the risk premium is not linearly related to IR. Therefore, the use of panel smooth transition regression analysis will result in more precise results.
When the RS is below 36.42%, noise traders’ risk premium will fall 0.72% for every 1% increase in their IS. Similarly, for every 1% rise in noise traders’ IS, a 1% firm size risk premium will lead to a 0.69% change in stock returns, the book-to-market ratio will lead to a 0.16% change, and the system risk will lead to a -0.52% change. When the individual IS are above 36.42%, then individual traders’ risk premium will fall 0.65% for every 1% rise in retail IS. For every 1% increase in the IS, the 1% firm size risk premium will lead to a 0.68% change in stock returns, the book-to-market ratio will lead to a 0.24% change, and the system risk will lead to -0.73% change. Other results for the study period from 2006 to 2013 are listed in Table 7.
Table-6. Marginal effects of the cost of capital regression
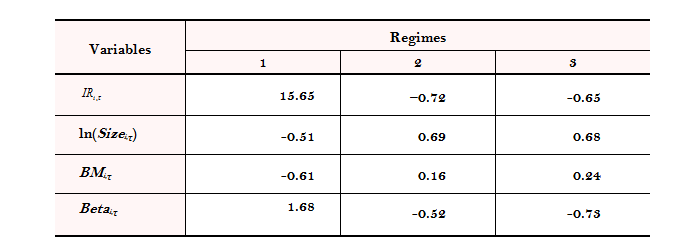
Note: IR denotes the idiosyncratic risk. ln(Size) denoting the market value and BM denoting the book-to-market ratio are the control variables. Beta denotes the market factor.
Table-7. Number of firms across different regimes, 2006-2013
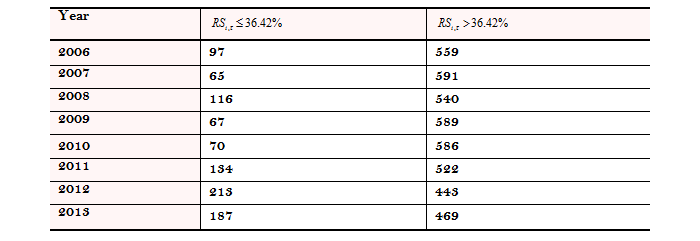
Note: RS denotes the retail investor sentiment. The threshold value of the retail investor sentiment is 36.42%.
5. CONCLUSION
The anomalies associated with IR in the literature are interesting research topics, and to date there has been no clear conclusion as to whether or not IR is positively related to stock returns. Based on investor decision-making under uncertainty, which is seen as a dual-classification process, this paper argues that the use of a more appropriate research method could lead to more convincing results. This paper thus applies panel smooth transition regression analysis to examine whether the relationship between IR and stock returns varies with the individual IS.
The empirical results indicate that noise traders require high (low) risk premiums when their shareholdings are high (low) IR. As a result, the IR is positively related to stock returns. The empirical results also show that IR is negatively related to risk premium. Noise traders with high (low) IS will require a low (high) risk premium. Therefore, retail IS is negatively related to stock returns. For a given idiosyncratic risk, retail traders with low sentiment require lower stock returns than traders with high sentiment. In other words, the relationship between idiosyncratic risk and stock returns varies with the retail traders’ sentiment.
This paper has the following implications for both investors and academic researchers. First, retail investors should pay more attention to investor sentiment, especially retail sentiment, because this will affect the stock returns when facing the same idiosyncratic risk. Next, for researchers, there appears to be a need to clarify the relationship between the idiosyncratic risk and stock return, and set up a theory (or model) to explain the stock return when considering both the idiosyncratic risk and the retail sentiment. One limitation of this work is the definition of retail sentiment and the frequency of idiosyncratic risk due to the proxies used in this work. It is thus suggested that more proxies of idiosyncratic risk and retail sentiment are used in the future research.
Funding: This study received no specific financial support. |
Competing Interests: The authors declare that they have no competing interests. |
Contributors/Acknowledgement: All authors contributed equally to the conception and design of the study. |
REFERENCES
Ang, A., R.J. Hodrick, Y. Xing and X. Zhang, 2006. The cross-section of volatility and expected returns. Journal of Finance, 51(1): 259-299.View at Google Scholar
Baker, M. and J.C. Stein, 2004. Market liquidity as a sentiment indicator. Journal of Financial Markets, 7(3): 271-299.View at Google Scholar | View at Publisher
Baker, M. and J. Wurgler, 2006. Investor sentiment and the cross-section of stock returns. Journal of Finance, 61(4): 1645-1680. View at Google Scholar | View at Publisher
Bali, T.G. and N. Cakici, 2008. Idiosyncratic risk and the cross section of expected returns. Journal of Financial and Quantitative Analysis, 43(1): 29-58. View at Google Scholar
Bali, T.G., N. Cakici, X. Yan and Z. Zhang, 2005. Does idiosyncratic risk really matter? Journal of Finance, 60(2): 905-929. View at Google Scholar | View at Publisher
Barberies, N., M. Huang and T. Santos, 2001. Prospect theory and asset prices. Quarterly Journal of Economics, 116(1): 1-53.View at Google Scholar
Barberis, N. and M. Huang, 2009. Preferences with frames: A new utility specification that allows for framing of risks. Journal of Economic Dynamics and Control, 33(8): 1555-1576. View at Google Scholar | View at Publisher
Brandt, M.W., A. Brav, J.R. Graham and A. Kumar, 2010. The idiosyncratic risk puzzle: Time trend or speculative episodes? Review of Financial Studies, 23(2): 863-899.
Brown, G.W. and M.T. Cliff, 2004. Investor sentiment and the near-term stock market. Journal of Empirical Finance, 11(1): 1-27.View at Google Scholar
Campbell, J.Y., M. Lettau, B. Malkiel and Y. Xu, 2001. Have individual stocks become more volatile? An empirical exploration of idiosyncratic risk. Journal of Finance, 56(1): 1-43. View at Google Scholar | View at Publisher
Chou, P.H., Y.Z. Zhang and M.C. Lin, 2007. The interaction between investor sentiment and stock returns. Review of Securities and Futures Markets, 19(2): 153-190. View at Google Scholar
Conrad, J.S., A. Hameed and C. Niden, 1994. Volume and autocovariances in short horizon individual security returns. Journal of Finance, 49(4): 1305-1329.View at Google Scholar | View at Publisher
DeLong, J.B., A. Shleifer, L.H. Summers and R.J. Waldmann, 1990. Noise trader risk in financial markets. Journal of Political Economy, 98(4): 703-738.View at Google Scholar | View at Publisher
Dennis, P. and D. Strickland, 2005. The determinants of idiosyncratic risk, Working Paper, University of North Carolina, Chapel Hill, 37.
Fama, E.F. and K.R. French, 1992. The cross-section of expected stock returns. Journal of Finance, 47(2): 427-486.View at Google Scholar
Fama, E.F. and K.R. French, 1993. Common risk factors in the returns on stocks and bonds. Journal of Financial Economics, 33(1): 3–56. View at Google Scholar | View at Publisher
Fisher, K.L. and M. Statman, 2000. Investor sentiment and stock returns. Financial Analysts Journal, 56(2): 16-23.View at Google Scholar
Fu, F., 2009. Idiosyncratic risk and the cross-section of expected stock returns. Journal of Financial Economics, 91(1): 24-37. View at Google Scholar
Gonzalez, A., T. Teräsvirta and D. Van Dijk, 2005. Panel smooth transition regression models. Research Paper Series No. 165. Quantitative Finance Research Centre, University of Technology, Sydney.
Gonzalez, R. and R. Wu, 1999. On the shape of the probability weighting function. Cognitive Psychology, 38(1): 129-166. View at Google Scholar | View at Publisher
Goyal, A. and P. Santa-Clara, 2003. Idiosyncratic risk matters! Journal of Finance, 58(3): 975-1008. View at Google Scholar | View at Publisher
Guo, H. and R. Savickas, 2008. Average idiosyncratic risk in G7 countries. Review of Financial Studies, 21(3): 1259-1296. View at Google Scholar | View at Publisher
Im, K.S., M. Pesaran and Y. Shin, 2003. Testing for unit roots in heterogeneous panels. Journal of Econometrics, 115(1): 53-74. View at Google Scholar | View at Publisher
Jiang, G.J., D. Xu and Y. Yao, 2009. The information content of idiosyncratic risk. Journal of Financial and Quantitative Analysis, 44(1): 1-28. View at Google Scholar
Kahneman, D. and A. Tversky, 1979. Prospect theory: An analysis of decision under risk. Econometrica, 47(2): 263-291. View at Google Scholar | View at Publisher
Lee, H.-I., H. Hsu, L.-K. Hu and C.-C. Lin, 2011. Portfolio insurance with ratcheted floor as a longterm asset management strategy: Implications of loss aversion. Applied Economics Letters, 18(15): 1449-1454. View at Google Scholar | View at Publisher
Levin, A., C.F. Lin and C.S.J. Chu, 2002. Unit root tests in panel data: Asymptotic and finite-sample properties. Journal of Econometrics, 108(1): 1-24. View at Google Scholar | View at Publisher
Malkiel, B.G. and Y. Xu, 2006. Idiosyncratic risk and security returns. Working Paper, Princeton University.
Phillips, P.C.B. and P. Perron, 1988. Testing for a unit root in time series regression. Biometrika, 75(2): 335-346. View at Google Scholar | View at Publisher
Rajgopal, S. and M. Venkatachalam, 2011. Financial reporting quality and idiosyncratic return volatility. Journal of Accounting and Economics, 51(1-2): 1-20. View at Google Scholar | View at Publisher
Shiller, R., 1984. Stock prices and social dynamics. Brookings papers on Economic Activity, 2(Fall): 457-498.View at Google Scholar | View at Publisher
Shiller, R.J., K.-Y. Fumiko and F. Yorshior, 1996. Why did the nikkei crash? Expanding the scope of expectations data collection. Review of Economics and Statistics, 78(1): 156-164. View at Google Scholar | View at Publisher
Shleifer, A. and L.H. Summers, 1990. The noise trader approach to finance. Journal of Economic Perspectives, 4(2): 19-33. View at Google Scholar
Tsai, P.J., Y.J. Wang and C.C. Chang, 2009. Investor sentiment, firm characteristics, and stock returns in Taiwan. Taipei Economic Inquiry, 45(2): 273-322. View at Google Scholar
Wei, S.X. and C. Zhang, 2005. Idiosyncratic risk does not matter: A re-examination of the relationship between average returns and average volatilities. Journal of Banking and Finance, 29(3): 603-621. View at Google Scholar | View at Publisher